Computer Vision for Vehicles
Under the pressure of increasing population, crowded traffic, the energy crisis, and environmental concerns, current transportation systems have run into serious challenges in the following respects: safety, security, efficiency, mobile access, and the environment [1] . There have been over 200,000 pedestrian fatalities in the last 30 years in US. Eighty percent of police reports [1] cited driver errors as the primary cause of vehicle crashes. With the availability of faster computers, better sensor technology, and wider coverage of the wireless communication network, Intelligent Vehicles and Intelligent Transportation Systems (ITS) are gradually being seen as a crucial innovation to improve safety and to reduce damages. It is estimated that implementing collision-avoidance systems in vehicles could prevent 1.1 million accidents in the US each year — 17 percent of all traffic accidents, which could save 17,500 lives and $26 billion in accident-related costs [2] . The demand for in-car electronic products is increasing. Around 35 percent of the cost of car assembly comes from electronics [3] .
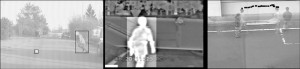
Figure 2: Segmentation results for urban day-time driving, for night driving, and for pedestrian tracking.
Environment-understanding technology is very vital to provide Intelligent Vehicles with the ability to respond automatically to fast-changing environments and dangerous situations. To obtain perceptual abilities, it is expected to automatically detect static and dynamic obstacles and obtain their related information, such as locations, speed, collision/occlusion possibility, and other dynamic current/historic information. Conventional methods independently detect individual pieces of information, which are normally noisy and not very reliable. Instead we propose a fusion-based and layered-based information-retrieval methodology to systematically detect obstacles and obtain their location/timing information for visible and infrared sequences. The proposed obstacle-detection methodologies take advantage of connections between different kinds of information and increase the computational accuracy of obstacle information estimation, thus improving environment-understanding abilities and driving safety. Three examples are shown in Figures 1 and 2. [4] [5] [6] [7]
References
- I.~T.~S. of~America, “National intelligent transportation system program plan: A ten-year vision,” the United States Department of Transportation, Tech. Rep., January 2002. [↩] [↩]
- The Intelligent Vehicle Initiative: Advancing “Human-Centered” Smart Vehicles. Available: http://www.tfhrc.gov/pubrds/pr97-10/p18.htm [↩]
- “Asia – New Hotbed for Consumer Automotive Electronics.” Available: http://www.technewsworld.com/story/52539.html [↩]
- Yajun Fang, Sumio Yokomitsu, Berthold Horn, Ichiro Masaki, “A Layered-based Fusion-based Approach to Detect and Track the Movements of Pedestrians through Partially Occluded Situations.” IEEE Intelligent Vehicles Symposium 2009 (IV2009). [↩]
- Fang. Y., Horn. B.K.P., Masaki I., “Systematic information fusion methodology for static and dynamic obstacle detection in ITS.” 15th World Congress On ITS, 2008. [↩]
- B.K.P.Horn, Y. Fang, I. Masaki, “Time to Contact Relative to a Planar Surface.” IEEE Intelligent Vehicles Symposium 2007. [↩]
- Y. Fang, K. Yamada, Y. Ninomiya, B.K.P. Horn, and I. Masaki, “A Shape-Independent-Method for Pedestrian Detection with Far Infrared-images.” Special issue on “In-Vehicle Computer Vision Systems” of IEEE Transactions on Vehicular Technology, Vol.53, No.6, Nov. 2004, pp.1679-1697. [↩]